Re-counting crime: New methods to improve the accuracy of estimates of crime (2020-2022)
(ESRC secondary data initiative)
There is probably no other scientific endeavour more relevant to the field of Criminology than to count crime accurately. Crime estimates are central to policy. They are used to in the allocation of police resources, and more generally they are a central theme of political debate with apparent increases in crime serving as an indictment on existing law and order policies. Academics also make regular use of crime statistics in their work, both seeking to understand why some places and people are more prone to crime, and using variations in crime to help explain other social outcomes. And of course, members of the public also refer to this information. For example, historic crime trends are now included on many house buying websites.
Currently, there are two main ways of estimating the amount of crime: directly using police records of incidents that they are aware of; and approximating crime using victimisation surveys like the Crime Survey for England and Wales, where a sample of people are asked to report any victimisations in the past year. Theoretical work has highlighted a number of sources of potential error in these data, suggesting that both approaches are deficient. However, we currently lack an empirically robust quantification of the difference sources of error in each. Nor do we fully understand the potential impact that these errors might have on the estimates from analyses that makes use of this data, although evidence from other fields suggests that this may be at a minimum substantial.
In this project we will use cutting edge statistical models developed in the fields of epidemiology, biostatistics and survey research to estimate and adjust for problems of measurement error present in police recorded crime and crime survey data. Drawing on data from 2011 to 2019 we will show the extent of systematic bias and random error in these two data sources, and how these errors may have evolved over time. Once the examination of the presence of measurement error in crime data is completed, we will use our findings to generate adjusted counts of crime across England and Wales, providing a unique picture of how different crimes vary across space and time. Finally, we will use these new crime estimates in tandem with ‘off the shelf’ measurement error adjustment techniques to demonstrate the potential influence that measurement error has on the findings of existing research.
Alongside this rigorous empirical work, we will also engage in a range of capacity building exercises to furnish researchers with the necessary skills to incorporate measurement error adjustments in their own work with crime data.
Work Package 1. Understand the measurement error mechanisms affecting crime data
The start of the project seeks to build a solid theoretical foundation to inform our subsequent empirical work. Through Work Package (WP) 1 we will provide a comprehensive literature review identifying the different types of systematic and random measurement error mechanisms affecting crime estimates. At least three forms of systematic errors and one form of random errors in reported crime rates can be anticipated. The latter is the least harmful, as it stems from the smoothing process undertaken to anonymise police records at the area level (Ceolin et al., 2014). More prominent sources of systematic error are incomplete ‘clearance rates’ (HMIC, 2014), under-recording (Roberts & Roberts, 2016), and those crimes that are never reported (Skogan, 1977) all of which might realistically be expected to vary across police forces and by crime type. These errors are likely to be substantial. HMIC (2014) warned that over 800,000 reported crimes go unrecorded each year (around 19% of all recorded crime). Skogan’s (1977) comparison of crime rates from police records to those from the first American National Victimization Survey showed that under-reporting was especially evident for less serious incidents (see also Hart & Rennison, 2003; Langton et al., 2012; Lynch & Addington, 2006). Moreover, cooperation with police services and crime reporting rates are conditioned by neighbourhood conditions, meaning police records are likely to be more valid in some areas than others (Bottoms et al, 1986; MacDonald, 2001; Tarling & Morris, 2010; Jackson et al., 2013).
Work Package 2. Combine crime survey estimates and police recorded crime counts
A key ‘innovation’ of this project stems from the conceptualisation of inconsistencies between police data and estimates from the Crime Survey for England and Wales as a measurement error problem. Doing so allows us to capitalise on new methodologies to improve our understanding of the measurement flaws in both police data and the CSEW (Oberski et al., 2017; Law et al., 2014), and to help us adjust for their biasing effect (Carroll et al., 2006; Gustafson, 2003). This is important, the more we understand about the nature and prevalence of measurement error problems in crime figures, the better equipped we are to undertake the necessary adjustments. In WP2 we will build on recent work which has demonstrated how Multi-Trait Multi-Method (MTMM) models can be used to augment administrative records with survey data to isolate the systematic and random forms of error, and estimate the ‘true value’ of an underlying outcome of interest (Oberski et al., 2017; Pyrooz et al., 2019; Yang et al., 2018). Treating the two sources of data as different methodological approaches and identifying a set of comparable offences within each dataset, it is possible to isolate bias (systematic errors) and variance (random errors) associated with the data collection from the shared variance observed in the two data sources across crime types. We can therefore directly quantify the extent and type of measurement error affecting each crime source.
Work Package 3. Generate bespoke adjustments and estimate corrected crime counts
Having estimated the nature and extent of measurement error in police recorded crime and CSEW estimates, WP3 will draw on methodological innovations stemming mostly from the field of biostatistics to develop ex-post adjustments for the ‘errors-in-variables’ (Fuller, 2009) problem affecting studies relying on either of these two forms of crime data. In particular, we will assess the utility of two existing approaches: SIMEX and Bayesian adjustments. Most adjustments assume a simple form of measurement error known as classical error (Novick 1966), X*=X+V, where X* is the observed variable, equal to the true variable X, plus a random measurement error term, V. Since the existing evidence suggests the dominant type of measurement error affecting crime rates calculated from police records is likely to be systematic – under-reporting proportional to the true crime rate – we will employ a modified form of the classical model, X*=XV. Setting the mean of the error within the (0,1) interval we can reflect a type of measurement error that is systematically negatively biased in a rate proportional to the true value . The specific distribution of V will be defined based on the estimations carried out in WP2 and will vary according to area (police force), period and offence. Further elaborations of these adjustments will also aim to account for additional forms of classical additive random errors identified in WP2.
Understanding mode switching and non-response patterns (2018-2019)
(Understanding Society Survey Methods Fellowships)
Abstract
The shift from single mode designs to mixed mode, where a combination of interview modes are used to collect data, is one of the key challenges of contemporary longitudinal studies. The decision about what modes to use and how to implement them can have a big impact, influencing costs, field-work procedures, non-response and measurement error.
The research proposed here aims to better understand one of the key characteristics of a mixed mode design: how people transition from one mode to another in time. This is essential for a number of reasons. Firstly, it can inform targeting strategies. For example, it can be used to target those people that are more likely to shift from a cheap mode to a more expensive one. It can also be used in models for dealing with non-response after data collection, such as weighting models. Thirdly, it can be used to explain measurement error that appears due to the mode design.
This paper will investigate the process of changing modes by looking at waves 5-10 of the UKHLS Innovation Panel. Latent class analysis will be used to find the underlying patterns of change in time of modes. The clusters found will be used both as dependent variables, to understand who are the types of respondents in each, and as independent variables, to predict future wave non-response and mode selection. Findings will inform the design and use of the main UKHLS study.
Estimating and correcting for multiple types of measurement errors in longitudinal studies (2017-2019)
(National Centre for Research Methods – Methodology Research project)
Abstract
Survey data, in its myriad forms, is essential in modern societies for policy development, business and marketing, as well as academic research. Longitudinal datasets (where the same individuals are interviewed on repeated occasions over time), are particularly important because they enable the analysis of ‘within-individual’ change which is increasingly recognised as essential for developing causal explanations of the social world. For example, longitudinal data have played a key role in understanding the impact of long term poverty on children’s educational and labour market prospects, the effect of smoking and alcohol consumption during pregnancy on child and parent outcomes, and on the factors underpinning intergenerational social mobility.
Yet even though the importance of longitudinal data is widely recognised, research on the quality and accuracy of longitudinal data is surprisingly sparse. Certain issues have been especially neglected, such as how to detect and correct for errors in the measurement of key concepts, so-called ‘measurement error’. Measurement error can be thought of as the difference between the conceptual ‘true’ score of an individual on a particular characteristic and what is actually measured in a survey. For instance, a particular survey respondent might actually weigh 81 kilos, yet in the survey data is recorded as weighing 80 kilos. There are a number of different reasons why the difference between the measured value and the true score might arise, which can be thought of collectively as different forms of measurement error. Measurement error is particularly problematic in longitudinal data, because it can incorporate multiple types of errors that appear at different time points. If such errors are present in a data set – and the existing research evidence suggests that their presence is widespread – then analyses and conclusions will be negatively affected. In short, we run the risk of drawing incorrect conclusions relating to key areas of public policy.
This proposal is for a project that will develop a new type of model to enable estimation of, and correction for, multiple types of errors in longitudinal data. This model will make it possible to estimate and correct simultaneously for:
- Method effects (where the response scale used for the question biases answers);
- Acquiescence (where respondents tend to agree to questions regardless of their content);
- Social desirability (where respondents provide answers in ways that are considered socially desirable);
- Cross-cultural effects (where measurement errors vary cross-culturally).
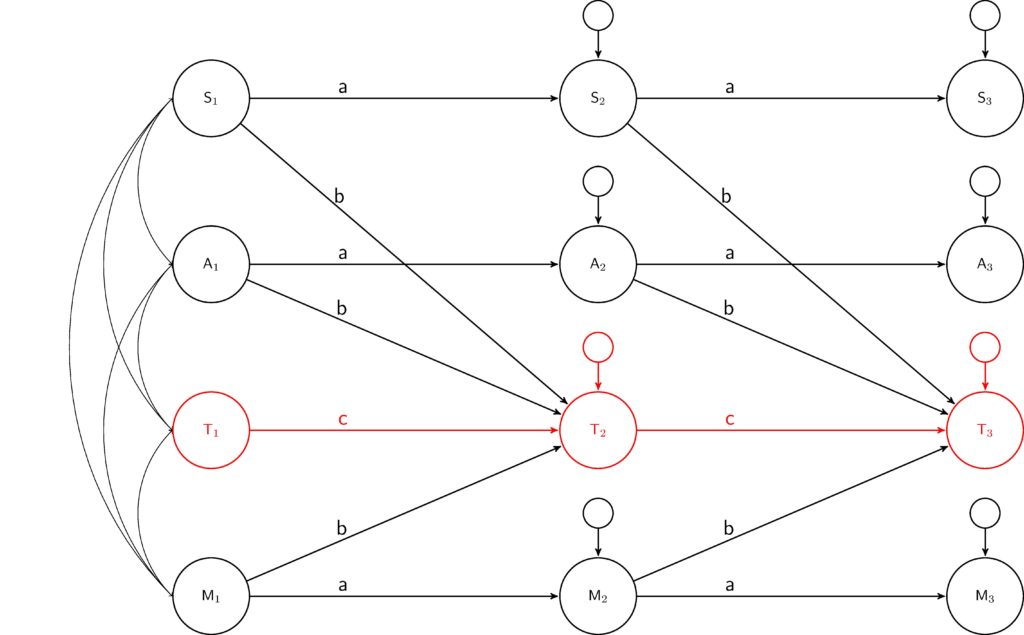
In addition to controlling for these different measurement errors the methods to be developed will also enable the evaluation of how they change over time. This will allow researchers to differentiate between measurement errors that are stable in time from those that are time specific, thus enabling powerful correction of error in longitudinal data. Additionally, the project will contribute to important debates in psychology, political science and survey methodology regarding the relationship between stable traits and errors in surveys. Similarly, the new model will enable researchers to estimate how the stability and changes in errors influence substantive survey answers in longitudinal data.
The project will also contribute to the increasingly prominent and controversial debate regarding public attitudes to immigration and immigrants, which will be the substantive focus of the analyses through which the new methods will be applied and tested. The impact of the project on this topic will be twofold: directly, through the substantive research on this topic and indirectly, through the improved measurement quality and by enabling secondary data users to run their analyses on the corrected version of the scale.
A simulation of the long term effects of an adaptive fieldwork design in longitudinal surveys (2018)
(National Centre for Research Methods – International Visiting Exchange – Melbourne Institute)
Abstract
Survey data continues to be essential for the work of social scientists. This is also true for longitudinal data where multiple measurements are taken at different points in time for the same respondents. Nevertheless, in recent years increasing costs and non-response have put pressures on survey agencies to improve the way they collect data.
One of the proposed solutions is called an adaptive design approach. This implies the division of data collection in multiple stages and applying different strategies for particular groups of respondent and stages that maximizes their propensity to answer and/or minimize costs. While this approach has great potential it nevertheless ignores the context of longitudinal studies. This is problematic as data collection in longitudinal studies have to consider at the same time both short term outcomes, such as participation at the current wave, as well as long term outcomes, for example participation in 5 years.
The present project aims to develop the framework of adaptive survey designs that accounts for both short term and long term outcomes in longitudinal studies. This will be done by developing a series of simulations that are based on data collected in the UK and Australia.
The research will help improve data collection in longitudinal studies, which is a major investment in the social sciences. It will also help improve the current literature in the adaptive survey literature and will enable more realistic decision making in data collection in general.